The advanced three-dimensional extension of high-efficiency video coding (3D-HEVC) is the latest coding standard for 3D video. The coding of the depth map for 3D-HEVC is very time-consuming. With the development of deep learning, it has become feasible to employ convolutional neural networks (CNNs) to predict the coding unit (CU) division of the depth map. However, there are three types of CU sizes: 64, 32, and 16, which makes it difficult to unify the model. The features of the depth map are very different from the texture map. In view of the aforementioned problems, we propose an adaptive CU size CNNs for fast 3D-HEVC depth map intracoding. We first employ spatial pyramid pooling to fully extract the features of the three types of CUs. Then, we apply the nonlocal self-attention mechanism to make it suitable for depth maps. Compared with the 3D-HEVC reference algorithm, the proposed network reduces the coding time by an average of 35.7%, while the quality degradation of the synthesized virtual view is negligible. |
ACCESS THE FULL ARTICLE
No SPIE Account? Create one
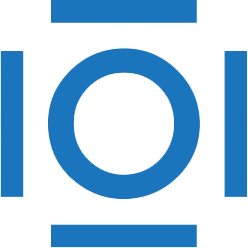
CITATIONS
Cited by 2 scholarly publications.
Copper
Volume rendering
Computer programming
Video
Video coding
3D modeling
Lithium