Image semantic segmentation is a fundamental problem in the field of computer vision. Although the existing semantic segmentation model based on fully convolutional neural network continuously optimizes the segmentation effect, the inherent spatial invariance of the network still leads to cause the loss of object edge details. Moreover, most models use the pixel-by-pixel loss to optimize the target, and the dependencies between pixels are ignored. When facing objects with smaller spatial structures in the image, the segmentation result is not satisfactory. Based on the theory of relative entropy and mutual information, we propose an overall objective loss function that integrates pixel similarity and image structure similarity. It can better pay attention to the structure and detail information of small objects in space by modeling the dependency relationship between pixels. We use the DeepLabv3+ network based on group normalization, with the improved ResNet50 as the backbone. After that, considering the particular advantages of superpixel segmentation for object edges, we propose a superpixel edge optimization algorithm, which combines pixel-level semantic features and superpixel-level regional information to obtain the semantic segmentation results after edge optimization. Experiments on PASCAL VOC 2012 and cityscapes datasets show that the proposed method improves the performance of semantic segmentation and shows better results in small target structures and object edge details. |
ACCESS THE FULL ARTICLE
No SPIE Account? Create one
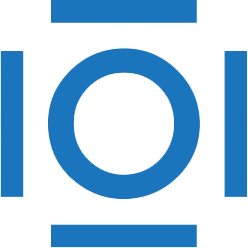
CITATIONS
Cited by 2 scholarly publications.
Image segmentation
Convolution
Image processing algorithms and systems
Optimization (mathematics)
Image information entropy
Convolutional neural networks
Image processing