We propose a method to change the shadows cast by vehicles based on unpaired data, which is used to solve the problem of insufficient datasets for target detection in autonomous driving scenarios. This method can increase the training samples of road vehicles in different time periods and reduce the time needed to make complex annotations of datasets. Direct use of generative adversarial networks with cycle-consistency constraints can achieve mapping transformation of unpaired domains, but it is difficult to completely learn the mapping between different shadow domains. To solve this problem, V-ShadowGAN is proposed. First, to train the model, we construct an unpaired dataset of vehicle shadows in different time periods. Second, the shadow mask extractor and the shadow mask discriminator are constructed to extract shadow masks and guide the generation of shadows in the network. Finally, the spatial attention mechanism is added to the generator, which improves the overall quality of the generated image by changing the channel weight of the network and deepening the network. Various experiments show that V-ShadowGAN can effectively change the vehicle shadow. |
ACCESS THE FULL ARTICLE
No SPIE Account? Create one
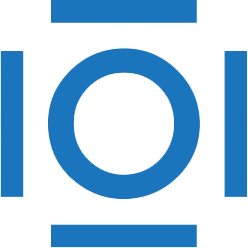
CITATIONS
Cited by 2 scholarly publications.
Image quality
Roads
Sun
Gallium nitride
Image processing
Data modeling
Visualization