We evaluated whether using synthetic mammograms for training data augmentation may reduce the effects of overfitting and increase the performance of a deep learning algorithm for breast mass detection. Synthetic mammograms were generated using in silico procedural analytic breast and breast mass modeling algorithms followed by simulated x-ray projections of the breast models into mammographic images. In silico breast phantoms containing masses were modeled across the four BI-RADS breast density categories, and the masses were modeled with different sizes, shapes, and margins. A Monte Carlo-based x-ray transport simulation code, MC-GPU, was used to project the three-dimensional phantoms into realistic synthetic mammograms. 2000 mammograms with 2522 masses were generated to augment a real data set during training. From the Curated Breast Imaging Subset of the Digital Database for Screening Mammography (CBIS-DDSM) data set, we used 1111 mammograms (1198 masses) for training, 120 mammograms (120 masses) for validation, and 361 mammograms (378 masses) for testing. We used faster R-CNN for our deep learning network with pretraining from ImageNet using the Resnet-101 architecture. We compared the detection performance when the network was trained using different percentages of the real CBIS-DDSM training set (100%, 50%, and 25%), and when these subsets of the training set were augmented with 250, 500, 1000, and 2000 synthetic mammograms. Free-response receiver operating characteristic (FROC) analysis was performed to compare performance with and without the synthetic mammograms. We generally observed an improved test FROC curve when training with the synthetic images compared to training without them, and the amount of improvement depended on the number of real and synthetic images used in training. Our study shows that enlarging the training data with synthetic samples can increase the performance of deep learning systems. |
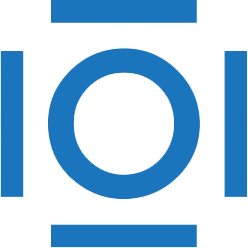
CITATIONS
Cited by 27 scholarly publications.
Mammography
Breast
Digital mammography
3D modeling
Data modeling
Monte Carlo methods
Detection and tracking algorithms