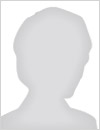
Reference histomorphometric data of healthy human kidneys are lacking due to laborious quantitation requirements. We leveraged deep learning to investigate the relationship of histomorphometry with patient age, sex, and serum creatinine in a multinational set of reference kidney tissue sections.
A panoptic segmentation neural network was developed and used to segment viable and sclerotic glomeruli, cortical and medullary interstitia, tubules, and arteries/arterioles in digitized images of 79 periodic acid-Schiff (PAS)-stained human nephrectomy sections showing minimal pathologic changes. Simple morphometrics (e.g., area, radius, density) were measured from the segmented classes. Regression analysis was used to determine the relationship of histomorphometric parameters with age, sex, and serum creatinine.
The model achieved high segmentation performance for all test compartments. We found that the size and density of nephrons, arteries/arterioles, and the baseline level of interstitium vary significantly among healthy humans, with potentially large differences between subjects from different geographic locations. Nephron size in any region of the kidney was significantly dependent on patient creatinine. Slight differences in renal vasculature and interstitium were observed between sexes. Finally, glomerulosclerosis percentage increased and cortical density of arteries/arterioles decreased as a function of age.
We show that precise measurements of kidney histomorphometric parameters can be automated. Even in reference kidney tissue sections with minimal pathologic changes, several histomorphometric parameters demonstrated significant correlation to patient demographics and serum creatinine. These robust tools support the feasibility of deep learning to increase efficiency and rigor in histomorphometric analysis and pave the way for future large-scale studies.Histological image data and molecular profiles provide context into renal condition. Often, a biopsy is drawn to diagnose or monitor a suspected kidney problem. However, molecular profiles can go beyond a pathologist’s ability to see and diagnose. Using AI, we computationally incorporated urinary proteomic profiles with microstructural morphology from renal biopsy to investigate new and existing molecular links to image phenotypes.
We studied whole slide images of periodic acid-Schiff stained renal biopsies from 56 DN patients matched with 2,038 proteins measured from each patient’s urine. Using Seurat, we identified differentially expressed proteins in patients that developed end-stage renal disease within 2 years of biopsy. Glomeruli, globally sclerotic glomeruli, and tubules were segmented from WSI using our previously published HAIL pipeline. For each glomerulus, 315 handcrafted digital image features were measured, and for tubules, 207 features. We trained fully connected networks to predict urinary protein measurements that were differentially expressed between patients who did/ did not progress to ESRD within 2 years of biopsy. The input to this network was either glomerular or tubular histomorphological features in biopsy. Trained network weights were used as a proxy to rank which morphological features correlated most highly with specific urinary proteins.
We identified significant image feature-protein pairs by ranking network weights by magnitude. We also looked at which features on average were most significant in predicting proteins. For both glomeruli and tubules, RGB color values and variance in PAS+ areas (specifically basement membrane for tubules) were, on average, more predictive of molecular profiles than other features.
There is a strong connection between molecular profile and image phenotype, which can be elucidated through computational methods. These discovered links can provide insight to disease pathways, and discover new factors contributing to incidence and progression.View contact details